Self-driving cars depend on various sensors to detect their surroundings. Although LiDAR and visual cameras receive most of the attention, radar is a vital piece of the jigsaw – albeit with some core drawbacks. With an intelligent software overlay on current instruments, Oculii hopes to reduce such shortcomings and give radar greater capability.
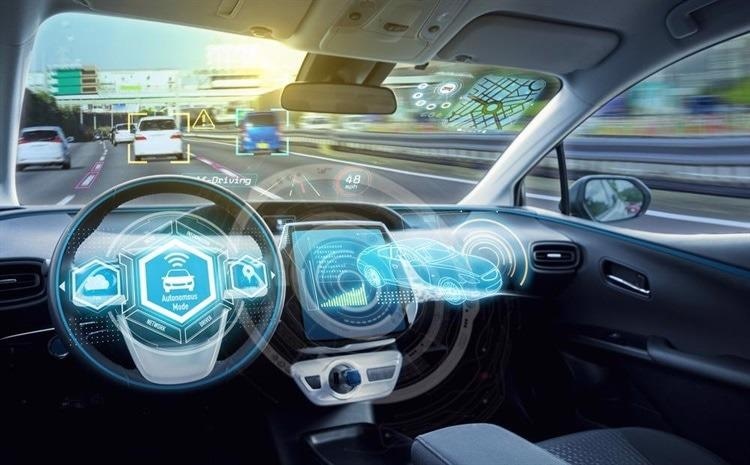
Image Credit: metamorworks/Shutterstock.com
Automotive Sensors Make Self-Driving Cars a Reality
Automobile radars, together with other sensory systems like LiDAR, ultrasonic sensors, and visual cameras, serve as the foundation for autonomous vehicles and advanced driver assistant systems (ADASs).
These technical developments result from highly complicated frameworks with a lengthy data processing route from sensors to controllers. Automobile radars identify objects and barriers, as well as their location and velocity in relation to the vehicle.
Autonomous vehicles are now a possibility because of breakthroughs in circuit architecture, which have been augmented by innovative signal processing techniques, artificial intelligence (AI), machine learning (ML), and computer-vision methods.
These vehicles also depend on various sensors, including a LiDAR, a camera, ultrasonic, a GPS, and radar sensors. Among these, radar provides the ability to observe vast ranges ahead of the automobile in low-visibility circumstances, which may aid in collision avoidance.
Basic Working of Radar
Radar detects obstructions by firing a focused beam of radio signals ahead of the car and receiving those signals back after reflection. This arrangement has remarkable range sensing accuracy and performs in practically any weather situation, plus radar systems are less expensive than LiDAR and other alternative techniques.
The benefits of radar include its better coverage and the ability of its radio waves to travel through small obstructions like raindrops, snow, and fog, making it critical for sensing the surroundings during adverse conditions.
These regular occurrences may completely confuse LiDAR and standard cameras, necessitating a backup system.
Oculii Addresses the Main Weakness of Radar Systems
The main drawback of radar is that, owing to the frequencies and how the antennae function, it cannot picture objects with high resolution in the same manner that LIDAR can.
Rather than distinct shapes, it shows fairly accurately positioned blobs. It still has useful qualities in a sensor package, but if improvements could be made to the quality of its results, it may be even better.
This is what Oculii achieves: it amplifies the abilities of a regular radar.
The organization says that turning over management of the radar system to its algorithms results in a 100x gain in spatial resolution. This allows for observations like something is, for example, two things close to one another rather than one big object, or that an entity is smaller than another nearby, or – with further calculation – that it is traveling in one direction or the other at a particular velocity in relation to the radar sensor.
Oculii is a revolutionary algorithm that tackles what has historically been thought of as a hardware challenge by dynamically increasing the spatial resolution of radar hardware systems by up to 100 times by employing an adaptable, phase-modulated waveform that varies instantaneously with the surroundings.
This novel algorithm makes self-driving cars a more commercially practical reality by allowing current radar technology to have much better quality. Radar technology is substantially less expensive than other 3D sensing equipment, and it has an established track record in the industry.
Its technology employs AI to alter the radar signals continuously in reaction to the surroundings. It is feasible to provide full 360° radar coverage for a range of as much as 350m irrespective of weather conditions by integrating four radar sensors at the front, back, and sides of the car.
Conventional Radars Vs. Radars with Oculii’s Virtual Aperture Imaging Software
A conventional radar waveform has one particular frequency and is non-adaptive and repetitive. Adding extra receptors is the only method to generate diverse waveforms.
The quantity of antennae determines the quality of classical radar systems. This implies that quality is restricted and that achieving better resolution requires additional gear - extra antennae, greater processing, bigger size, and more expenditures.
Adding antennae linearly improves performance but raises expenses, size, and power consumption exponentially, limiting the number of antennae that may be employed in a commercialized radar system.
The waveforms for Virtual Aperture Imaging (VAI) are phase modulated, dynamic and adaptive. At a particular instant, every receptor produces a distinct phase response. The signal is then interpolated and extrapolated to form a "Virtual Aperture".
Radar using Oculii's VAI algorithm improves sensitivity and resolution by utilizing artificial intelligence. That implies the radar is constantly improving as the algorithm scales exponentially with increasing amounts of data.
The adaptive VAI algorithm from Oculii delivers greater resolution with increased data and computing - growing in accordance with Moore's Law to offer a better definition and greater reach without compromising on affordability and cost-effectiveness.
The Oculii VAI algorithm is hardware-independent and incredibly customizable. It may be operated on just about any existing radar system to boost quality, giving a scalable perceptive solution ranging from ADAS to self-driving vehicles.
The Verdict
Self-driving car makers have yet to agree on a standard sensor package for autonomous vehicles, but technology like Oculii might provide radar a more important role.
Its shortcomings often cause it to be restricted to emergency brake sensing in front of the car or a similar circumstance, but with Oculii, Radar might play a greater part in autonomous vehicle decision-making mechanisms with more precision and information.
Continue reading: Trends in Sensor Technology and the Electric Vehicle Industry
References and Further Reading
Oculii (2021) AI Software Platform for Radar Perception.[online]. Available at: https://www.oculii.com/technology
Coldewey, D. (2021). Oculii looks to supercharge radar for autonomy with $55M round B. [online] TechCrunch. Available at: https://tcrn.ch/3xPGk7c
Just Auto (2021) GM makes Oculii investment to improve radar vision. [online] Available at: https://www.just-auto.com/features/gm-makes-oculii-investment-to-improve-radar-vision/
Patole, S. M., Torlak, M., Wang, D., & Ali, M. (2017). Automotive radars: A review of signal processing techniques. IEEE Signal Processing Magazine, 34(2), 22-35. Available at: https://ieeexplore.ieee.org/document/7870764
Disclaimer: The views expressed here are those of the author expressed in their private capacity and do not necessarily represent the views of AZoM.com Limited T/A AZoNetwork the owner and operator of this website. This disclaimer forms part of the Terms and conditions of use of this website.