Modern developments in precision tools such as precision machining tools and metrology instrumentation have realized extraordinarily high levels of precision. Thermal influences exist in all systems and tend to limit the achieved precision e.g. by causing thermal expansion.
In order to balance these effects, it is essential to determine the thermal impact. The current method to control thermal effects depends upon multi-parameter models. These models are obtained empirically, and are used to determine and forecast thermal impacts. The common parameters in these models are temperatures at different locations in the system and situational data e.g. power consumption of motor.
Some degreee of compensation for thermal effects can be achieved with this method. However, in certain applications, higher measurement precision and strength towards outer factors such as changes in ambient temperature is needed to get an acceptable compensation.
Challenges for Achieving High Accuracy
It is a challenge to accomplish higher precision levels with typical multi-parameter models because of the following reasons:
-
Non-linear temperature profile - Several temperature sensors are needed to reconstruct the temperature profile with adequate accuracy
-
Heat flow dynamics - The change in incoming or outgoing heat flow is unidentified as it is currently not established experimentally. A precise statement about whether the system is heating up or cooling down is quite difficult.
-
Temperature resolution – Basic temperature sensors have a limited temperature resolution, which hinders the exactness in measurement.
Expanding the system with supplementary temperature sensors accounts for only one of the problems mentioned. Moreover, the improvement in precision becomes smaller with each supplementary temperature sensor.
This article recommends the use of “heat flux” as an extra parameter, and reveals its advantages with regards to these three reasons. Heat flux (in W/m2) can be directly measured with a heat flux sensor (HFS).
Systems likely to benefit from heat flux sensors are listed below:
- Metrology systems
- Positioning systems
- Dosing systems
- Bonding systems
- Lithography
The probable benefits are illustrated with an example of the improvements gained by adding a single heat flux sensor to a high- precision metrology instrument (Figure 1).
.jpg)
Figure 1. Uncertainty in the prediction of mechanical deformations in a high precision metrology instrument. T = temperature, HFS = Heat Flux Sensor.
The measured and predicted mechanical deformations depend on a temperature-only model and a model with one heat flux sensor added (Figure 1). Whereas the method using only temperature sensors has a large ambiguous interval, adding one heat flux sensor to the system, improving the precision of the determined deformation value by a factor of 4. In the following article this finding is clearly explained, addressing the impact of a heat flux measurements covering all the above mentioned issues.
Although the illustration is based on a simplified machine element, the same recommendations can be applied to more complex geometries and systems. The block measured here is of uniform composition, W= 100mm wide, L= 300mm long, and T = 100mm thick. It is connected to a heat source on one end, which could for example symbolize a spindle in a milling machine.
Owing to the heat load, the block is heated with respect to its primary temperature T=20°C and a thermal expansion is induced which causes a displacement of the tool center point (TCP). By placing a heat flux sensor at the heated end of the beam, the quantity of heat exchanged via this surface is detected and available for the testing of the state of the system.
Simplified Simulation of Non-Linear Temperature Profiles
Before realizing a stable state, the temperature profile along any structural element is said to be in non-linear state. This is a fact for materials with high thermal resistances (Figure 2) for a mineral cast beam.
Using heat flux information, along with geometry and material properties, enables a better estimation of the temperature profile by affecting a neural network simulation. Therefore, complex thermal FEM simulations depending upon temperature-only information, can be cut down to manageable neural network models.
.jpg)
Figure 2. Left: Momentary temperature profile and thermal expansion in a block of mineral cast induced by a heat flux of 100W/m2. (Color scale shows temperature distribution along beam; thermal expansion is shown by exaggerated geometric deformation). Right: Temperature profile along center axis of beam. Due to the large thermal resistance of the material the temperature gradient is highly non-linear. Hence, thermal expansion (here approx. 12µm) is mainly concentrated at the hot end of the beam.
Improved temperature resolution
In order to establish the thermal expansion of a specific machine element, its mean temperature elevation (ΔTmean) above room temperature has to be detected. By placing temperature sensors along the beam, the temperature increase can be measured directly.
A typical resolution of a PT100 sensor is ±0.1K. This temperature resolution can be inadequate for high-precision compensation. Knowing how much heat has entered the beam, ΔTmean can be calculated from material properties and the geometry of the tool.
Basic heat flux sensors have a resolution of ±1W/m2. A copper block with heat flux resolution is used to calculate the mean temperature (Figure 3). By only considering the HFS resolution as a source of error, a resolution of ±10mK for the calculated ΔTmean is acquired. Radiation losses are taken into consideration in this example, which lead to an outgoing heat flow Qout.
.jpg)
Figure 3. Copper block with an incoming heat flux of 50 W/m2. ΔTmean is the temperature difference between the initial and the steady state of the simulation.
Reason 3 - Dynamic Measurement
By measuring the heat flux inside a system reveals immediately the amount of heat flowing and direction at the measurement location. Figure 4 shows an example based on the simple beam introduced above.
In the illustration, the heat source is a motor, which is operating at varied power levels in irregular intervals. The motor generates heat, which is partly absorbed by the beam and therefore causes a mechanical deformation.
There are two techniques to measure this effect. The first technique is to mount a temperature sensor at the beam surface to measure the temperature change ΔTmean induced by the heat flux. The second technique is to measure directly using a heat flux sensor. Although both the techniques provide essential data, the heat flux techniques provide extra insight into the state of the system.
When negative heat flows happens (i.e. heat is actually flowing back into the motor cooling), the measured temperature value ΔTmean is likely to indicate the same values as during heat up although the beam is cooling down. This effect happens due to a non-linear temperature profile. Consequently, heat flux provides a better understanding of dynamic effects and therefore allows for anticipatory compensation.
.jpg)
Figure 4. Top: Typical operation profile for a spindle motor. It is running at different speeds and switched on and off for several time periods. Part of the heat is dissipated into the supporting beam. Bottom: Heat flux and temperature curves caused by the operation profile shown in the top graph. Heat flux can even be negative (i.e. heat is actually flowing back into the motor cooling). The two encircled points in the temperature curve show, that although the temperature is the same, the heat flux in the system can differ greatly.
Conclusion
The article clearly reveals that the application of heat flux as an extra measurement parameter allows increase valuable data about a system under thermal influences. This is demonstrated with the use of heat flux sensors in some simple example setups. More complex systems will benefit even more from the measurement of heat flux than these simplified situations.
About greenTEG AG
greenTEG AG develops, manufactures and markets a new generation of heat flux and radiation sensors as well as thermoelectric generators. The company was founded as a spin-off from ETH Zurich in 2009. Since then greenTEG has won several awards for its technology and is currently supplying OEMs with customized sensor solutions and world-class researchers with measurement equipment for innovative experiments.
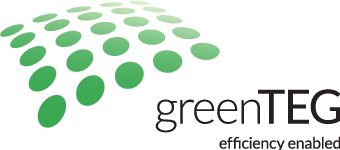
This information has been sourced, reviewed and adapted from materials provided by greenTEG AG.
For more information on this source, please visit greenTEG AG.