By taking touchscreen data collected by a learning application, researchers have laid out a possible framework for the improvement of 'virtual tutor' teaching tools. Digital education, the innovative use of digital tools and technology throughout the learning process, is on the rise, changing the way children and young adults are educated. The exploration of these digital platforms gives educators the opportunity to design more engaging learning material. In addition to this, such technology can also allow teachers and developers of curriculum and course materials to gauge how completely students are engaging with learning materials.
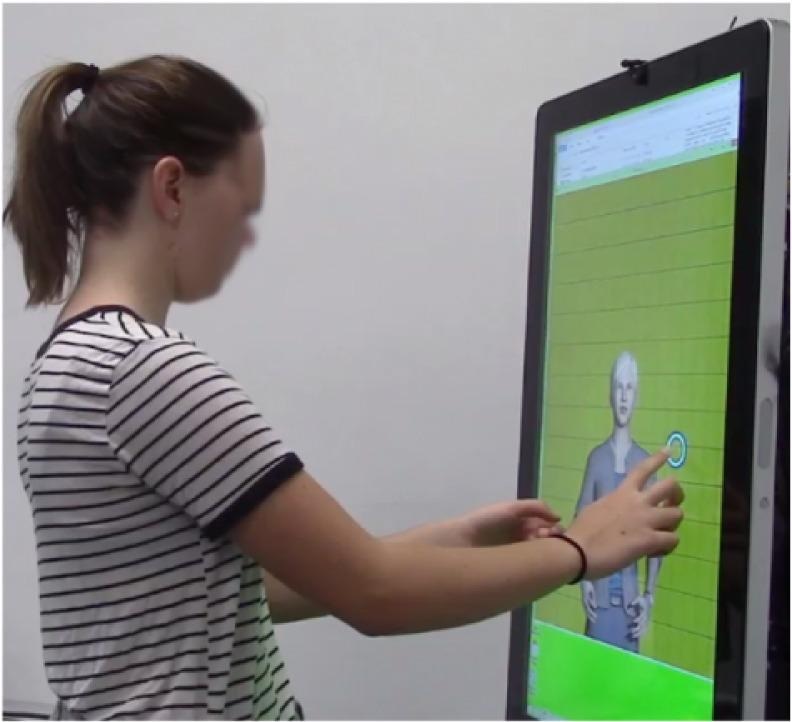
Image Credit: Pardos. Z. A., Rosenbaum. L. F., Abrahamson. D., [2021], 'Characterizing learner behavior from touchscreen data,' International Journal of Child-Computer Interaction, [https://doi.org/10.1016/j.ijcci.2021.100357]
Moreover, researchers are beginning to assess data collected by interfaces such as touchscreens and mouses to get a more complete understanding of not what we learn, but how we learn.
The real-time identification of student behaviors exceeds individual teachers' capacity, especially with class sizes continuing to grow. While Recurrent Neural Networks (RNNs) can be applied to modeling learner behavior, especially with online courses, the computer field is currently struggling to apply these systems to some kinds of data.
The question is, how do we best take advantage of the wealth of data that could potentially be delivered to us by a sea of touchscreens to make a more adaptive and individual digital learning experience?
A new paper published in the International Journal of Child-Computer Interaction¹ sets out the current situation regarding gathering such data and explains how this wealth of information can be processed. The authors, including Zachary A.Pardos, an Associate Professor at UC Berkeley studying adaptive learning and artificial intelligence (AI) at the University of California, Berkley, aim to describe a framework that could use student's behaviors and how they interact with course materials to develop an RNN that can refine digital learning platforms.
"A student who repeatedly deletes and re-types text into a free-response dialogue box may lack confidence in the subject matter, suggesting a need for greater support. A student who enters their responses quickly may need more challenging material," explain the authors. "Digital learning environments offer particular promise for flagging and supplying these pedagogical supports at scale if the data logs they produce can be accurately processed."
Taking Learning Data Out of Proportion
In their study, the team of researchers explored different techniques to identify patterns in student's touchscreen focusing on how to pick out signals from digital sensors that could be translated into useful information.
In order to do this, the team used an RNN to predict strategies employed by students and detect patterns in touchscreen data delivered while they interacted with a mathematics tutoring program called Mathematics Imagery Trainer for Proportionality (MITp). The data obtained was collected as part of a project that aims to create a virtual tutor that can automate the teaching of children.
MITp is an application that helps students understand the concept of proportion by having them move markers up and down on a touchscreen to signal the desired height ratio. The application is already being used by human operators to assess the learning strategies employed by small groups of students. If the app is to be used to do the same for larger groups, however, that will require a system that can automatically detect such strategies.
The team assessed the data with two models, one based upon touchscreen telemetry data that predicted student strategies. The second sought to better understand touchscreen behavior patterns as students changed in response to challenges posed by MITp. While the latter used a 'classic' RNN, the former relied upon an augmentation called long short-term memory (LSTM), which can classify patterns based on longer sequences.
The models were trained using a group of 49 students. Of these, five were labeled and run through the model with hidden states identified and visualized. These visualizations were then regularized by data from all 49 students and used to synthesize patterns of behavior.
The team aimed to improve this virtual tutor's abilities by training it to identify what strategies are being used by individual learners. This means more than registering what moves the student is making and understanding how they are making those moves. The team rationalizes that it will give the agent a window into the learner's thought processes enabling it to provide much more effective and individualized guidance.
Can LSTM-augmented recurrent neural networks (RNNs) get an A+ in education?
The researchers found that the application of LSTM-augmented RNNs to the touchscreen data used resulted in a moderate improvement in the classification of student performance. The model achieved over 47% accuracy in predicting the strategy being used by individual students from moment to moment compared to a success rate of around 40% achieved by a system with less advanced features.
When the classification technique switched from predicting strategies from moment to moment to a more general prediction of the strategies that would be employed by a particular learner, the accuracy of the RNN system jumped up to over 86%.
Interestingly, the team may have learned more from the system's failures than they did from its impressive successes. Where modeling failed to predict strategy, the researchers suggest that what the system was actually doing was not failing to spot good learning strategy, but rather, correctly identifying bad learning strategies. They labeled these gaps as 'dark spaces' in their modeling.
These behavioral patterns that do not directly solve a problem may indicate a critical 'stepping stone' to transition to a successful problem-solving approach, which could be a key element in our learning process.
"If this conjecture bears out, it would suggest integrating the models themselves into the software's real-time interaction regimen to illuminate for instructors — whether human or artificial — the dark matter of learning," the authors conclude.
Even with the improvements made by the LSTM model, predicting strategies is still a significant challenge. The authors suggest that this is because learning is a highly individualistic process making it tough to model with generalizable qualities.
One thing the LSTM augmented RNN did show itself to be particularly adept at was spotting a signal that could be used by the virtual tutor to predict that the learner is ready to move on to more challenging exercises.
The authors suggest that future testing could incorporate eye-movement tracking leading the tutor from an understanding of what a student is thinking to how a student is thinking. They also posit that a larger set of labeled students help could improve accuracy in classification and warrant a deeper integration of the models tested into a virtual tutor system.
Disclaimer: The views expressed here are those of the author expressed in their private capacity and do not necessarily represent the views of AZoM.com Limited T/A AZoNetwork the owner and operator of this website. This disclaimer forms part of the Terms and conditions of use of this website.
Source:
¹ Pardos. Z. A., Rosenbaum. L. F., Abrahamson. D., [2021], 'Characterizing learner behavior from touchscreen data,' International Journal of Child-Computer Interaction, [https://doi.org/10.1016/j.ijcci.2021.100357]