Artificial intelligence (AI) and machine learning have become increasingly prominent with the widespread availability of generative AI tools such as ChatGPT and DALL-E. One such industry is rail operations, facilitated by data collected via the Internet of Things (IoT).
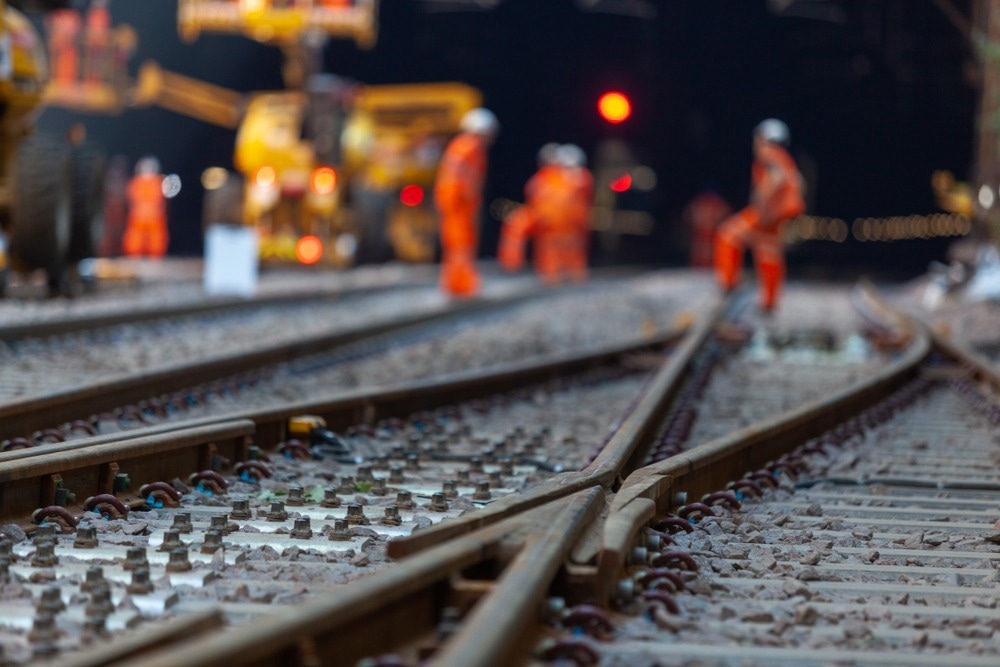
Image Credit: Matthew Nichol/Shutterstock.com
IoT refers to wirelessly interconnected sensors, monitoring stations, and other physical devices capable of sending or receiving information. AI can utilize the massive quantity of data collected during train and train network operation via the IoT, and spotting ways to improve the system. This article will discuss how AI and the IoT are being used to monitor rail networks and optimize their operation.
How Can AI Predict Maintenance Requirements?
One example of the potential usefulness of combining AI and the IoT is monitoring track vibrations using simple but robust accelerometers with internet capability. Track vibrations can be indicative of track failure, and thus be used to monitor where and when maintenance is required. However, inferring track failure from vibrations only is not as simple as measuring the magnitude, as differing trains, track arrangements, and environmental conditions could produce vastly differing vibration profiles.
A company named Konux, based in Munich, Germany, has collected billions of train traces over the last decade and correlated this data with maintenance information using AI over the vast German train network. This level of data interpretation allows for the prediction of failures in the system based on past events, thus informing where predictive maintenance should be performed. By utilizing an extensive IoT, with sensors placed numerously at key locations, an idea of the health of the system can be realized that surpasses current systems.
Furthermore, actively operating AI and IoT setups can respond to the ongoing condition of rail tracks and incoming vehicles, avoiding dangerous situations and providing information relating to speed and weight conditions. Ultimately, this technology could be used to actively inform train operators of probable issues, and dynamically adjust speed or route.
Currently, several thousands of these sensing devices are installed on railways across Germany, and have also been adopted in China, India, and Japan, each of which are investing heavily in an expanded or more finely optimized rail network. Similarly, Network Rail, the company that manages rail infrastructure in the United Kingdom, also extensively utilizes IoT-connected devices to monitor the rail network, detect maintenance issues, and perform predictive maintenance, totaling half a petabyte of data per week as of 2021.
Other sources of data utilized by Network Rail include aerial imagery and 3D LiDAR captured by drones, scheduling information, and footage collected by inspection trains, all of which are fed into AI to generate actionable predictive maintenance schedules.
How Can AI Optimize Route Planning and Scheduling?
Konux also utilizes AI to optimize train timetabling, network usage monitoring, and inspection planning, as have other industries such as truck and ship freight. A vast quantity of operator time and fuel is wasted with poor route planning and arrival scheduling, wherein the delay in unloading the present arrival slows subsequent arrivals.
These factors have been considered and planned for since the inception of the freight industry in any form, though the wide availability of precise location and time data, using modern GPS and the IoT, has allowed unparalleled assessment levels. As in many other fields to which AI has been applied, the huge quantity of data available is almost useless to human operators owing to the vast scale; thus, AI is exploited in combing through this data to highlight patterns and trends.
Interestingly, sources of data other than freight routes themselves may be useful in their optimization and may originate from nature. For example, in a 2020 paper published in Logistics, an ant colony model is utilized to optimize for multiple pickup and delivery routing by heterogeneous fleets, to minimize travel costs while considering real-world constraints such as driver working time.
These types of simulations and mathematical models, wherein vehicles follow a specific set of instructions for delivery and pickup while under certain constraints, are extremely useful in spotting trends in large and complex systems, where the ultimate result of fundamental behavioral changes can be interpreted.
Conclusions
Trains are already one of the most optimal forms of passenger and freight transport in terms of infrastructure cost and energy consumption, though they are severely bottlenecked by the existing size and arrangement of the trail network. Track and train maintenance, therefore, further exacerbate this bottleneck, and severely limit the consistency and reliability of the network.
Optimization of existing railway infrastructure is an extremely attractive and cost-effective way of expanding rail capacity, particularly in countries that are already saturated with rail. Where there is still room for rail network expansion, AI can be used to plan routes optimally, accounting for route popularity, cost of construction, topographical features of the terrain, and other factors that assist engineers when designing infrastructure.
It is important to note that while AI and the IoT assist in identifying problems that would only otherwise have been noted by foot inspection, the number of jobs affected should be minimal, as by optimizing the system, workers can be placed where they are most needed, and savings passed onto further expanding and improving the network.
References and Further Reading
https://www.squaredtech.co/konux-scaling-ai-iot-for-railway-optimization
https://iot.eetimes.com/uk-network-rail-uses-iot-ai-and-deep-learning-to-improve-the-worlds-oldest-railway-system/
Ky Phuc, P. N., & Phuong Thao, N. L.. (2021). Ant Colony Optimization for Multiple Pickup and Multiple Delivery Vehicle Routing Problem with Time Window and Heterogeneous Fleets. Logistics, 5(2), p.28. doi.org/10.3390/logistics5020028
Disclaimer: The views expressed here are those of the author expressed in their private capacity and do not necessarily represent the views of AZoM.com Limited T/A AZoNetwork the owner and operator of this website. This disclaimer forms part of the Terms and conditions of use of this website.