Hyperspectral imaging is an imaging technique that utilizes the detection of a very large region of the electromagnetic spectrum. Hyperspectral imaging relies on the use of two-dimensional focal plane imaging detectors in combination with diffraction gratings to separate the different wavelengths of light before they are focused onto the detection array.1
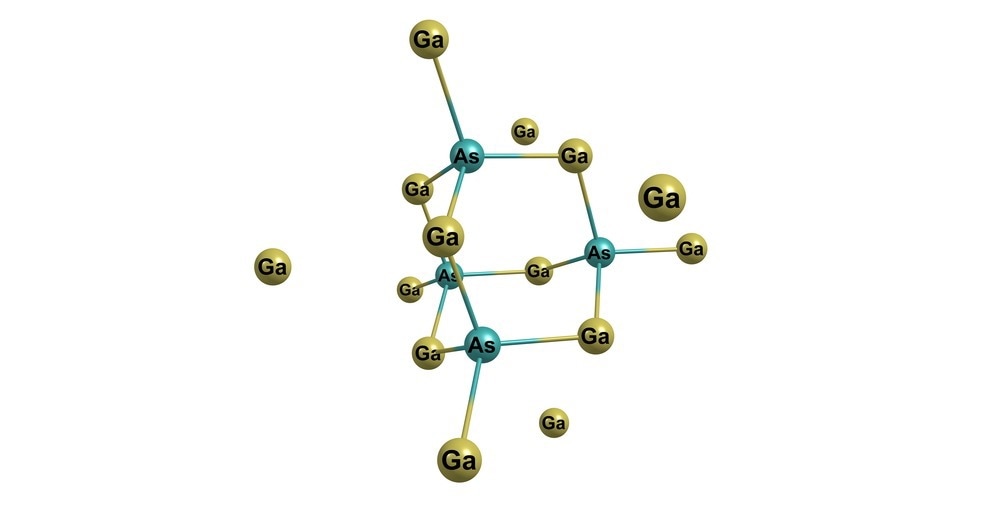
Image Credit: Igor Petrushenko/Shutterstock.com
The two-dimensional image captured in the array has one axis that corresponds to the wavelength of light being detected and the other to the position on the slit that was being imaged, which allows for the spatial resolution of the object being mapped.
The images obtained in different regions of the electromagnetic spectrum are usually grouped into what are known as bins or bands. More bins mean a higher spectral resolution but often less total signal per bin. A large number of bins also increases the volume of data to be processed, so there are a number of trade-offs between acquisition conditions and either on- or off-chip data bining or processing to be considered for optimal imaging.2
Some key applications of hyperspectral imaging include agriculture and terrain mapping, as well as art and heritage analysis.3,4 As well as the exact imaging conditions, though, another key consideration when choosing a hyperspectral imaging instrument is the combination of the wavelength range for operation and corresponding detector choice.
Importance of InGaAs Detectors in Hyperspectral Imaging
Many applications of hyperspectral imaging, particularly those for biological samples or devices working in low light conditions, benefit from working in the infrared region of the electromagnetic spectrum.5,6 Most standard CMOS or CCD imaging arrays work optimally in the visible region of the electromagnetic spectrum out to ~ 1100 nm.
Indium gallium arsenide (InGaAs) detectors are often the technology of choice for performing longer wavelength detection. InGaAs photodiodes often have detection ranges between ~ 800 – 2600 nm (covering most of the near IR and shortwave IR regions) by using a combination of different absorbing elements in a single detector.
Depending on the bandgap of the materials used in the detector, this detection range can be altered, but InGaAs detectors offer good detection efficiency across most of this range. Efficient detection is essential for reducing acquisition times and giving cameras a good sensitivity for operation in low-light conditions.
Applications of InGaAs in Hyperspectral Imaging
There are now a variety of InGaAs detector technologies available, including PIN photodiodes and avalanche photodiodes, which typically offer greater sensitivity. Such photodiodes can be combined into arrays for hyperspectral imaging applications.
To improve the range of applications InGaAs detectors can be used for, there have been great efforts to try and reduce the cost associated with the detectors, which has historically been quite high.7 Now, InGaAs detection arrays are being used in hyperspectral imaging devices for quality control of materials8 and devices have become sufficiently compact that InGaAs-based instruments can be used for remote sensing.
As hyperspectral imaging can be a non-destructive technique and certain infrared wavelengths can be used to ‘see through’ objects such as packaging or layers of paint, InGaAs detection technologies have also proved popular in cultural heritage studies as well for explosive detection and security applications. There are few alternatives for achieving the same level of detection efficiency in this region of the electromagnetic spectrum.
Advantages of InGaAs Technology
As well as being non-destructive and allowing for the imaging of ‘hidden’ objects, infrared detection also has the advantage of not requiring external illumination of the source. All objects that are above absolute zero in temperature will have an emission of thermal radiation, which is in the infrared region of the electromagnetic spectrum and so can be detected using InGaAs detectors.9
No requirement for external illumination makes InGaAs technologies highly appealing for remote sensing and machine vision applications as it means hyperspectral imaging can be performed in any type of weather or illumination conditions. For remote imaging, it may not be practical to have any control over the environment being imaged, so infrared detection may be more robust than visible hyperspectral imaging. Short-wavelength infrared has good atmospheric penetration as well, further improving the robustness of the imaging process.
While the optical set-ups for hyperspectral imaging were historically very bulky with multiple lens stacks and diffraction components, compact InGaAs detectors and improvements in the optical design have helped make miniaturized hyperspectral devices.10
Challenges and Future Directions
There are still some challenges when covering very wide wavelength ranges for InGaAs detectors, which is normally achieved by using combinations of photodiodes. Future work is to make single monolithic InGaAs photodiodes that integrate GaAs layer devices capable of simultaneous IR and visible detection, which would be highly significant for hyperspectral imaging.
Cost is still somewhat of an issue and the vulnerability of the detectors to both thermal fluctuations and radiation damage. Good progress is being made on the reduction of the amount of spectral cross-talk in hyperspectral imaging detectors and there are now a number of commercial solutions available.10
References and Further Reading
- ElMasry, G., & Sun, D. W. (2010) Principles of Hyperspectral Imaging Technology. Hyperspectral Imaging for Food Quality Analysis and Control, pp. 3–43. doi.org/10.1016/B978-0-12-374753-2.10001-2
- Zeegers, M. T., et al. (2020) Task-driven learned hyperspectral data reduction using end-to-end supervised deep learning. Journal of Imaging, 6(12). doi.org/10.3390/jimaging6120132
- Lu, B., et al. (2020) Recent advances of hyperspectral imaging technology and applications in agriculture. Remote Sensing, 12(16), pp. 1–44. doi.org/10.3390/RS12162659
- Wu, T., et al. (2017) Shortwave Infrared Imaging Spectroscopy for Analysis of Ancient Paintings. Applied Spectroscopy, 71(5), pp. 977–987. Retrieved from http://opg.optica.org/as/abstract.cfm?URI=as-71-5-977
- Manolakis, D., et al. (2019) Longwave infrared hyperspectral imaging: Principles, progress, and challenges. IEEE Geoscience and Remote Sensing Magazine, 7(2), pp. 72–100. doi.org/10.1109/MGRS.2018.2889610
- Akbari, H., et al. (2011) Cancer detection using infrared hyperspectral imaging. Cancer Science, 102(4), pp. 852–857. doi.org/10.1111/j.1349-7006.2011.01849.x
- Colin, T., et al. (2022) Development and integration of new functions in InGaAs imaging. Proc. SPIE Infrared Technology and Applications XLVIII, 12107, p. 5. doi.org/10.1117/12.2618921
- Mahesh, S., et al. (2015) Hyperspectral imaging to classify and monitor quality of agricultural materials. Journal of Stored Products Research, 61, pp. 17–26. doi.org/10.1016/j.jspr.2015.01.006
- Hashagen, J. (2015) Seeing Beyond the Visible in machine vision. Optik&Photonik, 3, pp. 34–37. https://onlinelibrary.wiley.com/doi/epdf/10.1002/opph.201500021
- Saute, B., et al. (2022) Next-generation thermal infrared hyperspectral imaging sensors. Proceedings of SPIE, 12094, p. 9. doi.org/10.1117/12.2619046
Disclaimer: The views expressed here are those of the author expressed in their private capacity and do not necessarily represent the views of AZoM.com Limited T/A AZoNetwork the owner and operator of this website. This disclaimer forms part of the Terms and conditions of use of this website.